AI-driven quality inspection: offloading data to reduce waste
In manufacturing, quality control is an essential part of assuring customer satisfaction and reducing scrap and waste. New technologies – like cloud computing, machine learning and computer vision – pave the way for higher speed and accuracy – and even quality predictions. delaware expert and data science solution lead Wouter Labeeuw shares his insights.
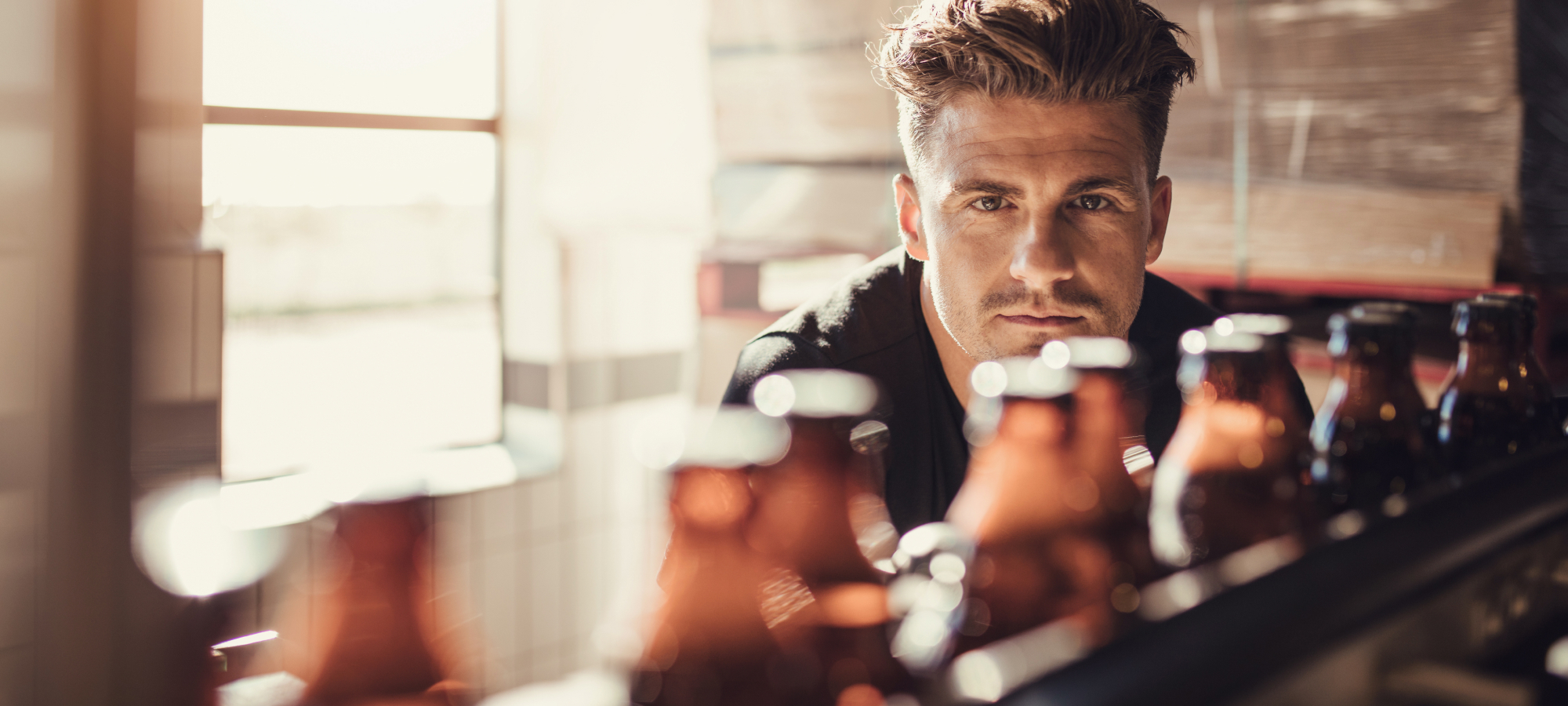
“We’re currently working on a major project to analyze the impact that environmental factors, like the weather, can have on product quality,” says Wouter. “For example, when atmospheric pressure increases, the pressure a production machine exerts could inadvertently increase as well. If we can train a machine-learning model to accurately predict and quantify that impact, we could make changes on the fly to prevent bad product batches. In time, production machines could even adjust themselves automatically to adapt to changing conditions.”
Offloading historical data from the shop floor to the cloud
For that to become a reality, however, a lot of pieces need to come together. “First and foremost, we need to find a way to efficiently collect and store all the historical data that’s needed to adequately train the model,” explains Wouter. “Many companies still store this data on premise in a server located on the shop floor. However, this is also where the operational data – which is needed for everyday operations – is stored. So, tinkering with it is extremely risky.”
Since it’s not recommended to risk compromising your operational data, the first step is to offload your historical data to a cloud environment. Wouter: “There are a lot of advantages to this approach. Number one, it’s less expensive than investing in hardware or buying additional shop floor licenses, since you only pay for what you use. Secondly, resources are virtually unlimited in the cloud: you don’t need to worry about maxing out capacity. And finally, it allows you to experiment with data analysis without worry. Of course, all of this taking into account important topics like bandwith and security to provide a stable and secure solution while respecting total cost of ownership.”
Gaining the right insights for impactful process improvements
For their latest project, Wouter and Axel orchestrated the data transfer and integration using Data Factory, Azure’s hybrid data integration service. The next step in the process, the actual analysis, is done using Microsoft Power BI and Databricks. But how does a data scientist determine which queries are worthwhile? “That’s one area where the client’s experience with their own processes and our technical expertise can truly complement each other,” explains Wouter. “Often, they already know – intuitively or by experience – which production parameters and environmental factors influence one another. We then provide the tools and the knowledge needed to analyze this interaction, quantify that impact, and, if possible, act on it to optimize the process.”
But first, data scientists like Wouter need to evaluate the results of each query to find out which analyses produce useful results – and which are not worth pursuing further. Wouter: “This evaluation process is done manually. The ultimate goal is, of course, to let the algorithm make decisions and even perform on-the-job adjustments. Before that can happen, however, you need to be absolutely sure that you’ll get the desired outcome.”
Data maturity is up, costs are down – it’s time to get started
While AI-driven quality control – where models are trained with historical data – has been around for a while now, there aren’t many practical implementations in Belgium yet. The main reasons for this, according to Wouter, are an ingrained belief that big data solutions are extremely expensive and not worth the trouble, and – up until recently – the lack of solid data governance. But the tide is slowly turning.
“We are noticing that a lot of companies are now catching up on data maturity,” says Wouter. “They are also seeing more and more evidence of success in AI-driven quality control from industry peers. And as they acquire more knowledge, they start to understand that these kinds of solutions aren’t actually that expensive at all, since you only pay for the cloud computing resources you use.”
“Another side effect of this rise in data maturity is that companies want to be able to perform data analyses themselves. That's why, at delaware, we focus on ‘enablement’ instead of ‘delivery’. We want to provide clients with all the tools and guidance they need to be successful.”
Interested in leveraging the power of AI to reduce waste and improve product quality? Download our e-book on efficieny in operations and discover how three domains of machine-learning technologies – computer vision, natural language processing and intelligent devices – can be applied in real business situations to add measurable value.